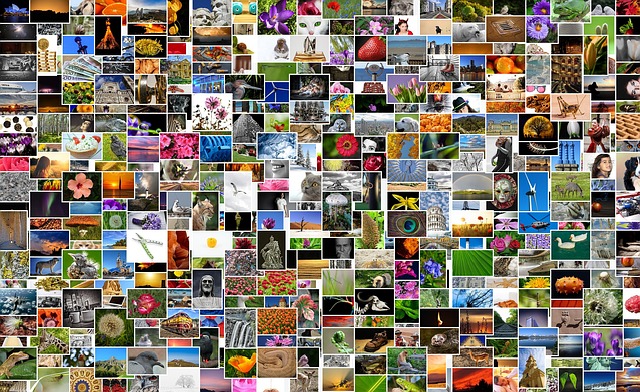
A First Look at Vector Sets
Last week, Redis announced vector sets—a new data structure coming in Redis 8. They also released Redis 8 RC1, which means we get to play with this new feature right now. And that’s exactly what we’re going to do!
We’ll explore vector sets through a real-world example: a user’s photo album. I picked this example because it shows off where vector sets really shine. Why does it work so well? Stick around to the end to find out.
Before We Get Started
Two big caveats before we dive into the details:
- Even though they are part of Redis 8, vector sets are in beta. They could change or even be removed from future versions of Redis. So, think about that before you ship code to production.
- This post isn’t intended to be an introduction to vectors, vector search, and embeddings. I’m going to assume that you have at least an inkling of what these things are. If you don’t, watch this talk that I gave at Jfokus where I explain it in detail.
Disclaimer delivered. Now, on with the fun!
What’s a Vector Set?
A vector set is a lot like other sets in Redis like the sorted set or the plain old set. However, a vector set contains labeled points in a multi-dimensional space—think X-Y coordinates but with more axes. The labels themselves are just simple strings. The points are a series of numbers—the coordinates in the multi-dimensional space. These numbers are the vector.
I can add vectors to a vector set using the VADD command. In this example I’m adding 512-dimensional embeddings of photos for a user named Alice. The photoId
is being used as the label and the embedding
returned from the call to embedPhoto
are the vector:
async function addPhotoToVectorSet(userId: string, photoId: string, pathToPhoto: string) {
const embedding: number[] = await embedPhoto(pathToPhoto)
const key = `user:${userId}:photos`
const values: string[] = embedding.map(v => v.toString())
const dims = embedding.length.toString()
await redis.sendCommand(['VADD', key, 'VALUES', dims, ...values, photoId])
}
await addPhotoToVectorSet('alice', 'photo:42', '/photos/IMG_0042.png')
await addPhotoToVectorSet('alice', 'photo:23', '/photos/IMG_0023.png')
await addPhotoToVectorSet('alice', 'photo:13', '/photos/IMG_0013.png')
Note that I am using TypeScript here and Node Redis, but this should translate nicely to whatever tool you’re using. Vector sets are so bleeding edge that the client libraries don’t even support them yet. So, we have to use the lower-level .sendCommand()
function which accepts a string[]
. Your client library of choice will have some variation of this.
Also, the .embedPhoto()
function is, effectively, pseudocode. Your magic embedding code goes in there. If you want to take a peek at that magic, check out my meme twin finding example.
So, a vector set is—surprise—a set of vectors. In most real-world use cases, those vectors will be embeddings, like the ones you’d generate from an image, a chunk of text, or even audio.
Using a Vector Set
Once you have vectors in a vector set, you can manipulate them:
const key = `user:${userId}:photos`
// removes a photo from the set
await redis.sendCommand(['VREM', key, 'photo:42'])
// the cardinality of the set
await redis.sendCommand(['VCARD', key])
// the number of dimensions the vectors in the set have, 512 in our case
await redis.sendCommand(['VDIM', key])
This isn’t exactly complex code—it just invokes the commands and the comment tells you what they do. There are lots of other commands as well. The full details for all of them are in the docs which I encourage you to peruse.
The command at the heart of vector sets is VSIM—it lets you search for vectors that are the most similar to a provided one. There are two main ways that you provide one—by value and by element.
Providing a vector by value is simply providing the vector as part of the command to Redis. This is the classic semantic search use case:
async function searchPhotos(userId: string, query: string): string[] {
const embedding: number[] = await embedText(query)
const key = `user:${userId}:photos`
const dims = embedding.length.toString()
const values: string[] = embedding.map(v => v.toString())
return await redis.sendCommand(['VSIM', key, 'VALUES', dims, ...values, 'COUNT', '3'])
}
Providing a vector by element is just using an existing vector in the vector set, referenced by its label. This is more of a recommendation pattern:
async function similarPhotos(userId: string, photoId: string) {
const key = `user:${userId}:photos`
return await redis.sendCommand(['VSIM', key, 'ELE', photoId, 'COUNT', '3'])
}
And, you can see how these might work nicely together:
// returns photos matching the query
const foundPhotoIds: string[] = await searchPhotos('alice', 'Show me pictures of animals')
// returns photos similar to the first found photo
const firstFoundPhotoId = foundPhotoIds[0]
const similarPhotos: string[] = await similarPhotos('alice', firstFoundPhotoId)
And that’s pretty much it for the code - at least enough for you to get started.
So What?
So, what’s the big deal? I could do all of this with the Redis Query Engine, right?
Absolutely—and in many cases, Redis Query Engine is still the right choice. It supports hybrid search, rich document indexing, and can scale across shards in a Redis cluster. If you’re doing full-text search, filtering on structured data, and searching across millions of vectors, Redis Query Engine is the way to go.
Vector sets offer something different. They’re simpler, easier to work with, and perfect for use cases where data is naturally scoped—to a user, a device, a region, or a moment in time. You don’t need to define a schema. You don’t need to manage an index. You just add vectors and search them.
Of course, this simplicity comes with trade-offs. Vector sets are all about the vector—that’s their focus. It’s in the name!. You won’t get rich document indexing or hybrid queries. JSON-based filtering is supported, but it’s not as flexible as what Redis Query Engine provides. And scaling across a cluster? Absolutely possible, but you’ll have to manage that in code yourself.
So while there’s overlap in what you can build, the way you build it—and the trade-offs you make—are different. It’s not about better or worse. It’s about using the right tool for the job.
Conclusion
If your application needs rich filtering, hybrid search, and global indexing, Redis Query Engine is the clear choice. But if you’re building lightweight, fast, and highly scoped similarity search—especially for things like user-specific data or ephemeral sessions—vector sets can be a great fit.
That’s why the photo album example works so well. Each user has their own vector set, which naturally avoids clustering issues and performance bottlenecks. You can quickly find similar photos, search by description, and build real-time recommendations—all without the overhead of Redis Query Engine.
Vector sets are still in beta, but they’re already looking like a powerful addition to the Redis toolbox. I’m definitely going to keep tinkering—seeing where they work and where they don’t.
Got ideas? Weird use cases? Built something cool? If you have, tag me on Bluesky, LinkedIn, or X. I’d love to see what you’re doing with vector sets.
—